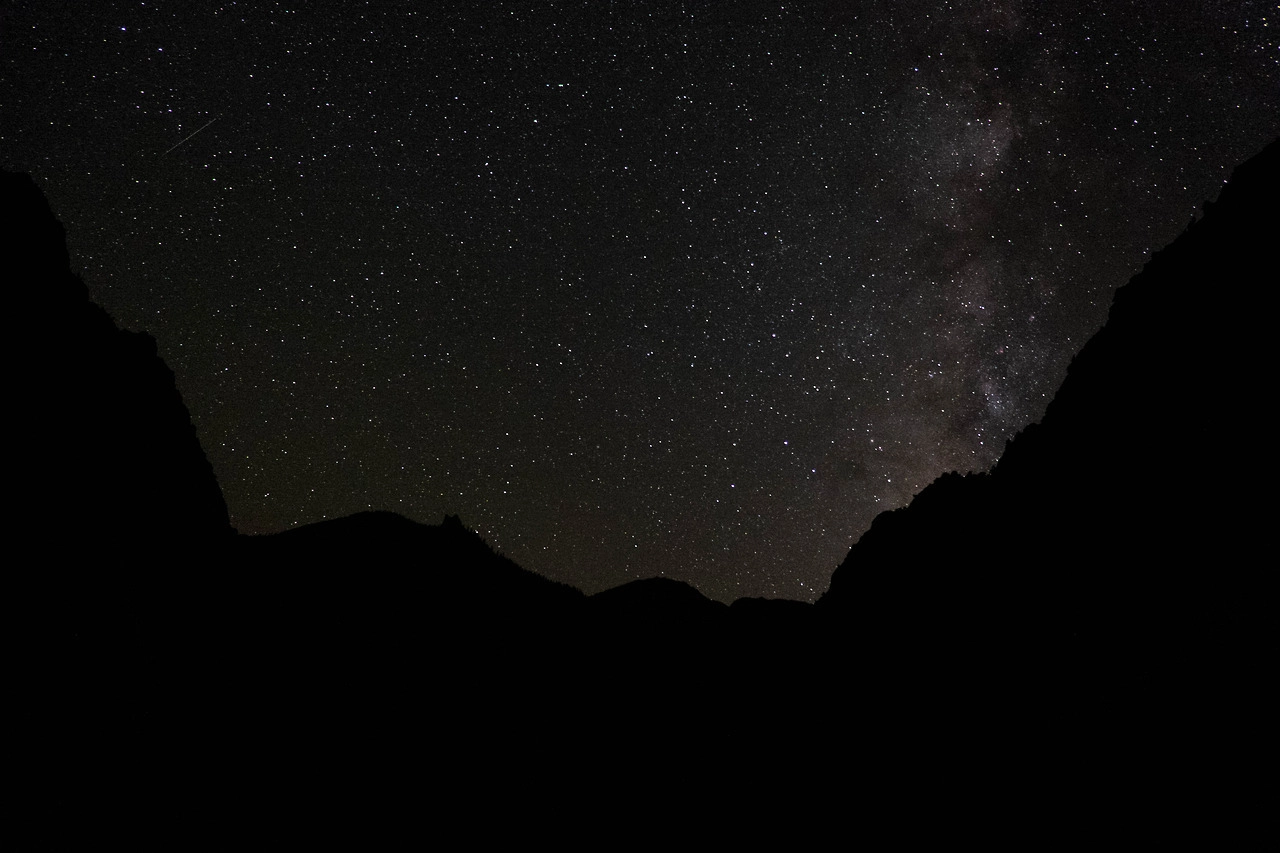
emulating interior stellar structure for binary population synthesis
Understanding the internal structure of stars is crucial to modeling their evolution. The novel binary population synthesis code POSYDON includes a module for interpolating stellar and binary properties at the end of binary MESA evolution. I developed a new emulation method for predicting stellar profiles, i.e. the internal stellar structure of density, hydrogen mass fraction, and helium mass fraction along the radial axis using machine learning techniques. We use principal component analysis for dimensionality reduction, and neural networks to make predictions. By delivering more information about the stars during evolution, these methods will expand the kinds of studies possible with POSYDON and other population synthesis codes, especially with respect to constraining uncertain evolutionary stages.
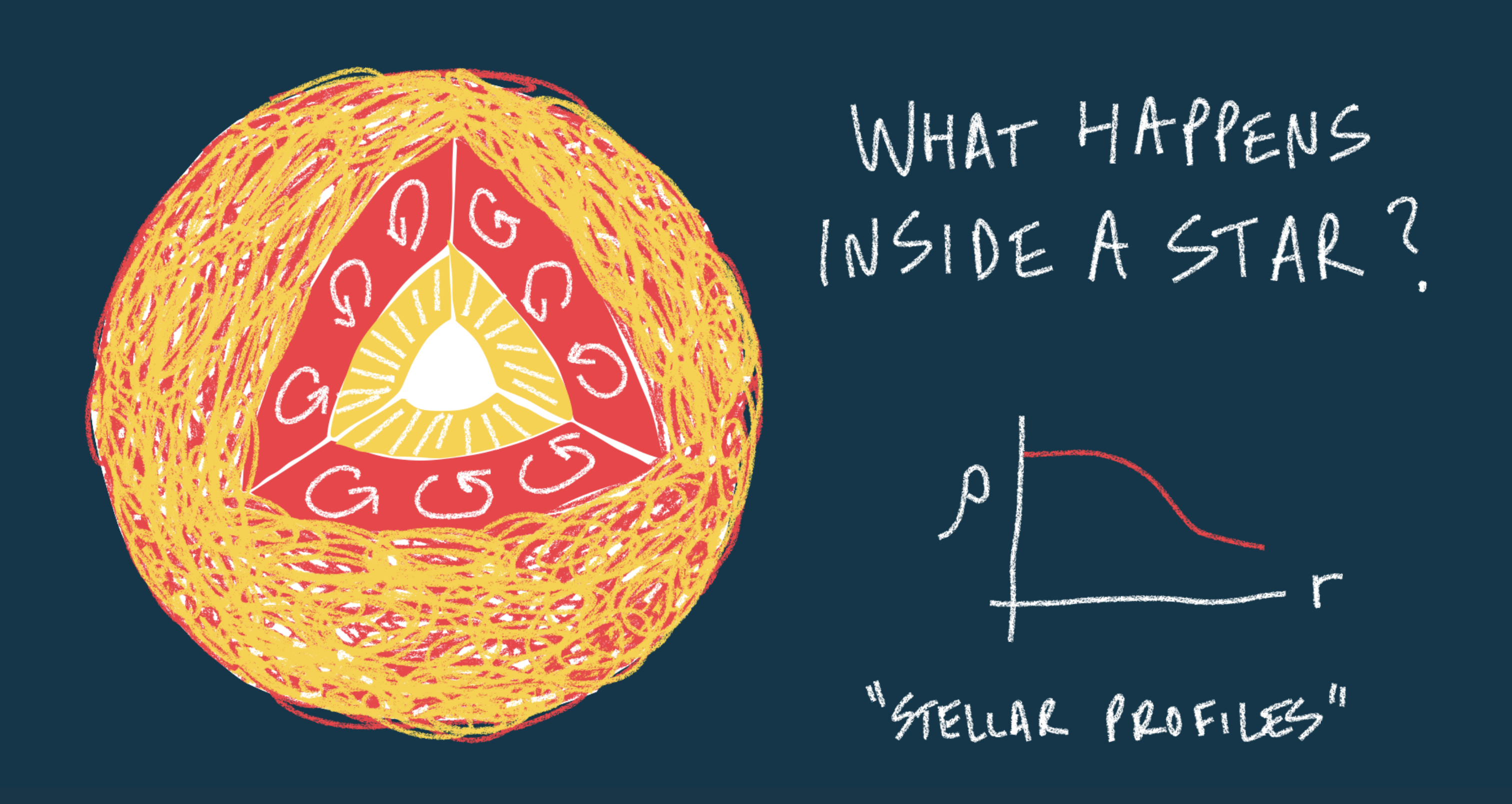
past work: emulating protoplanetary SEDs from a radiative transfer model
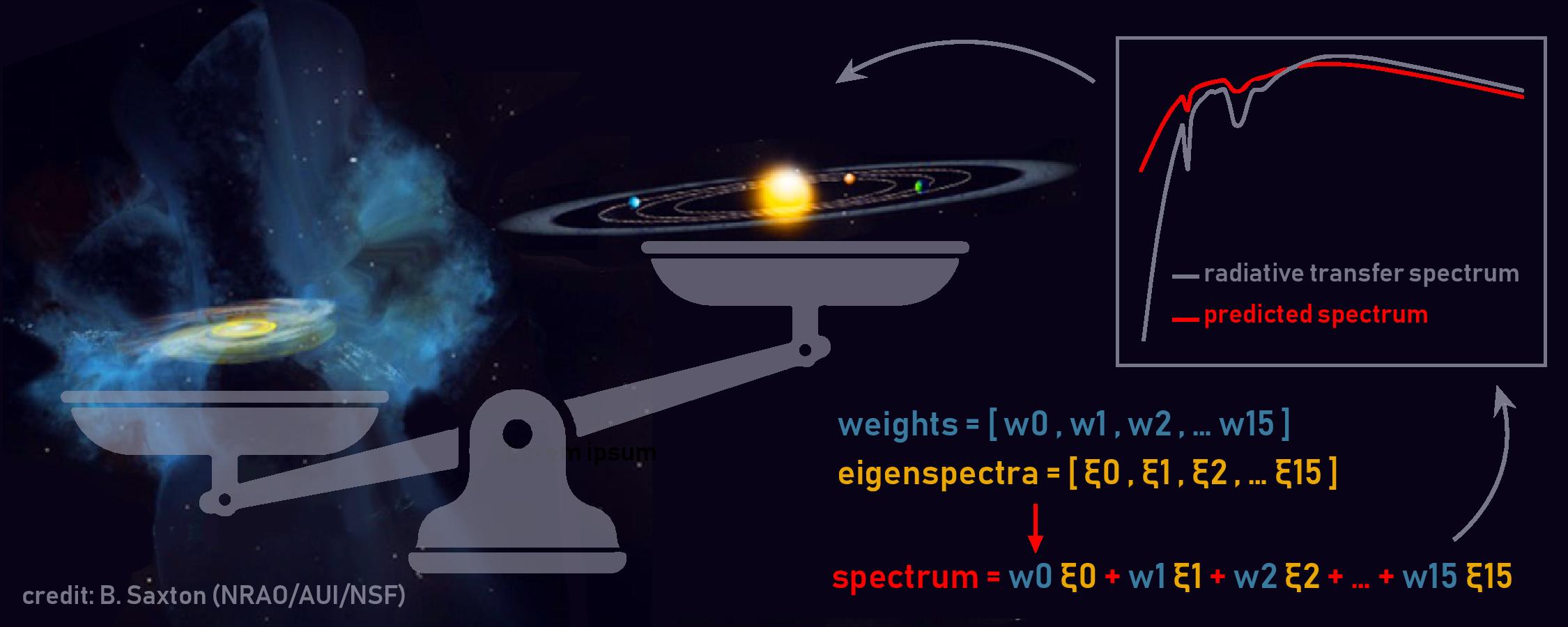
Current disk masses of Class II protostars, directly retrieved from dust masses, are too low to explain observed exoplanet populations. Pursuing masses of younger Class I disks could resolve this discrepancy, but they are still embedded in dusty envelopes, which complicates direct retrieval models. Using radiative transfer to forward model SEDs (spectral energy distributions) against observed disks for mass inference is effective but computationally expensive (Sheehan+ 2017)
I developed a surrogate model that efficiently emulates multi-wavelength continuum SEDs generated by an existing protoplanetary disk radiative transfer model. This model was designed as a forward model against observations, including incoming ALMA data, to achieve thorough disk mass estimates with fully propagated uncertainties for Class I protostars. This will help address one of the most prevalent questions in protoplanetary disks by resolving disk masses with observed exoplanet observations, and establish initial mass budget constraints for planet formation.
This work was advised by Dr. Ryan Loomis (NRAO) and Dr. Patrick Sheehan (Northwestern U). This project was funded by the NSF through the REU program at the NRAO. Additional support was provided by the NSF through award SOSPA7-004 from the NRAO.
past work in pulsar timing
I worked with Professor Andrea Lommen at Haverford College on noise reduction for x-ray pulsar timing data with the broad goal of enabling a gravitational wave detection using pulsars. For one project I gathered and analyzed thousands of observations from NASA’s Neutron Star Interior Composition ExploreR (NICER) mission to facilitate a statistical comparison of two different data filtration methods, which cut out observations based on geography and photon count-rate, respectively.
In another project, I applied Markov Chain Monte Carlo for Bayesian inference to comparatively model chromatic and achromatic time-dependent (red) noise spectra for pulsar timing data. Red noise in pulsars comes from a variety of sources, including dispersion measure changes in the interstellar medium (ISM), intrinsic spin noise, and a stochastic background of gravitational waves. I combined x-ray data from NICER with radio data to isolate electromagnetic frequency dependent (chromatic) components of the noise spectrum of PSR B1937+21. By observing the same pulsars in both the X-ray and radio bands, we are able to isolate which elements of the noise spectra come from interactions with the ISM. This will allow us to separate intrinpsic noise from what could be evidence of a gravitational wave detection.
Support for this work was provided by the NSF through the NANOGrav Physics Frontier Center
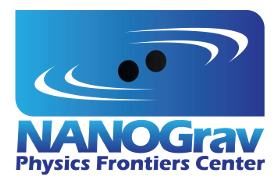
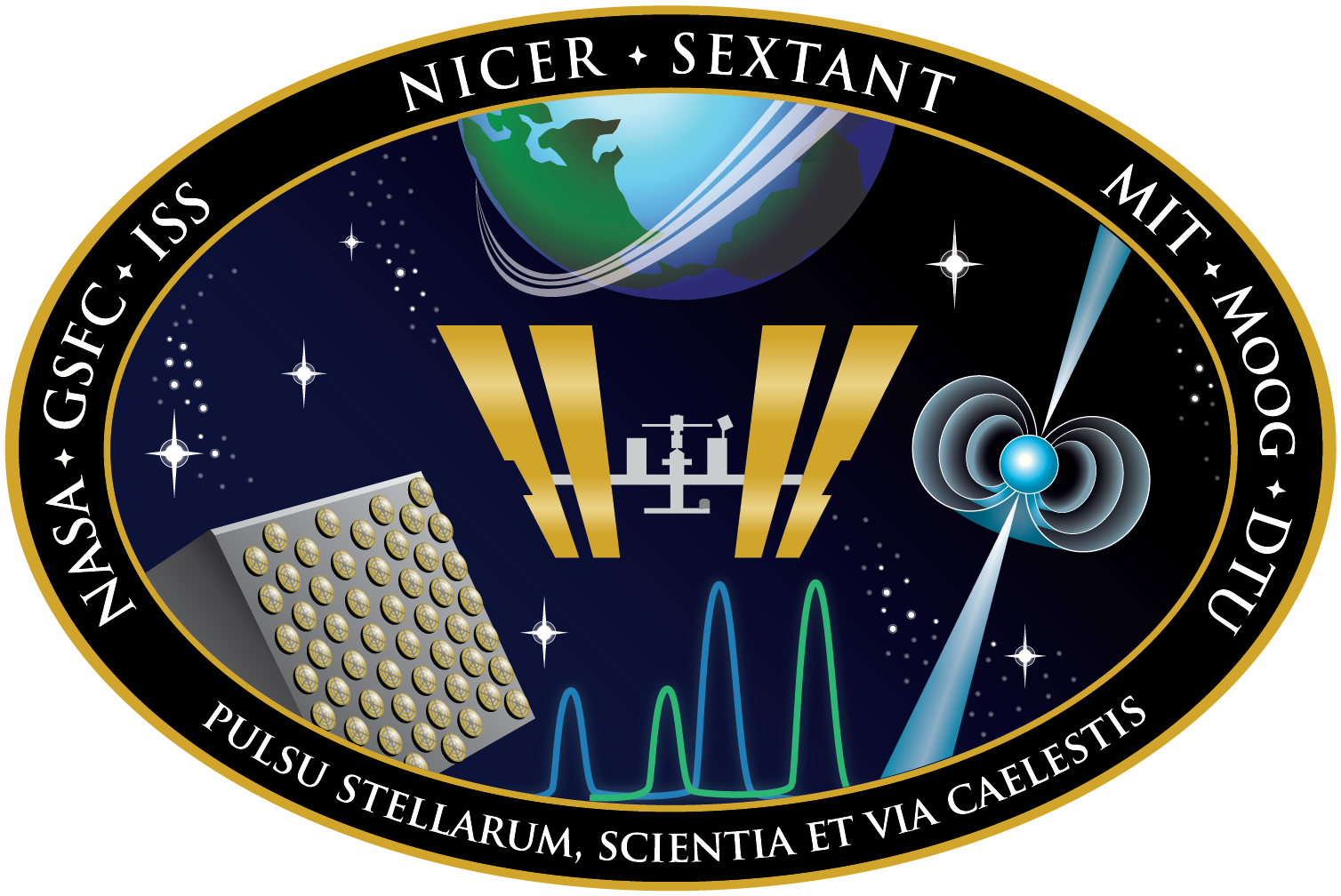